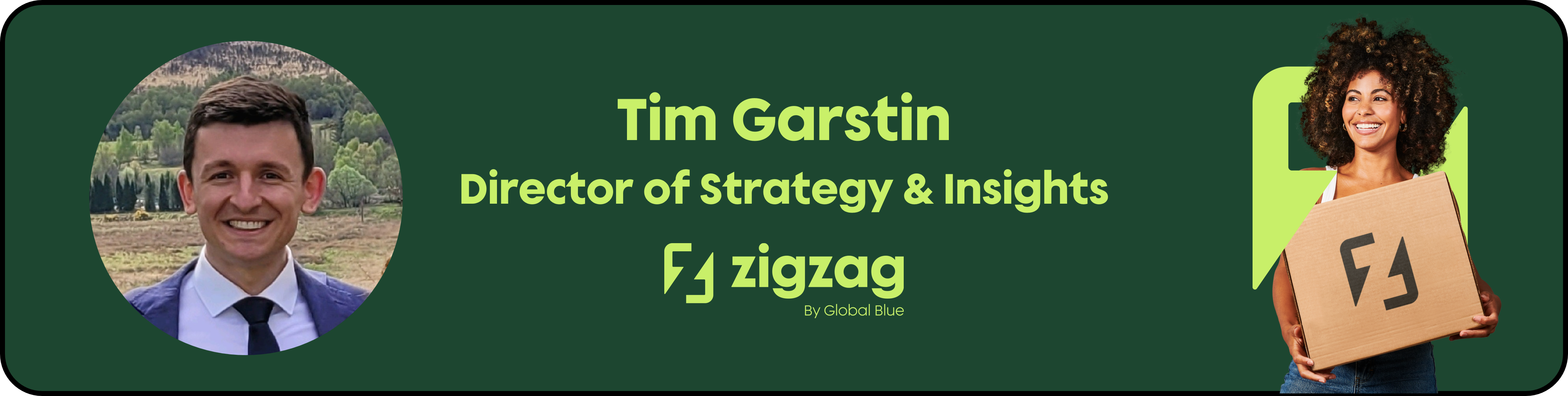
In a world of easy online shopping, returns are often seen as a challenge or hassle. Emerging AI powered eCommerce offerings are now starting to transform the customer experience and improve how we shop, exchange, and return to drive future business growth.
With the rise of AI and the maturing of predictive machine learning (ML) technologies, which are a subset of AI, retailers are now able to transform returns into opportunities and go further than ever before. AI and machine learning algorithms are focusing on driving improved customer return experiences that reduce overheads, drive efficiencies and automated decision-making that in turn improve customer loyalty.
1. Automated Carriage Routing Optimisation
Retailers face a big challenge to access the data that allows them to get to grips with their domestic and global returns. Without this, it is a challenge to optimise their carriage offering for returns to make informed decision-making.
That’s where smart carrier optimisation machine learning models come in. These models are designed to rank carrier route recommendations to the specific retailer preferences, such as on route reliability, transit times, route carriage costs, and support costs.
Recommendation algorithms are allowing retailers to transition to a more reliable service, with faster transit times, a lower average customer service costs, a lower cost carriage service or a more sustainable route. These models can learn and adapt recommendations to make sure that the retailers preferences stay updated, relevant, and effective to their needs.
Looking ahead, these models will likely transform the market further by enabling automated decision-making on re-routing based on the retailer's needs. This will include near real-time data to account for variable factors like short-term carrier performance degradation, weather patterns, and factoring in seasonal issues with individual services and routes.
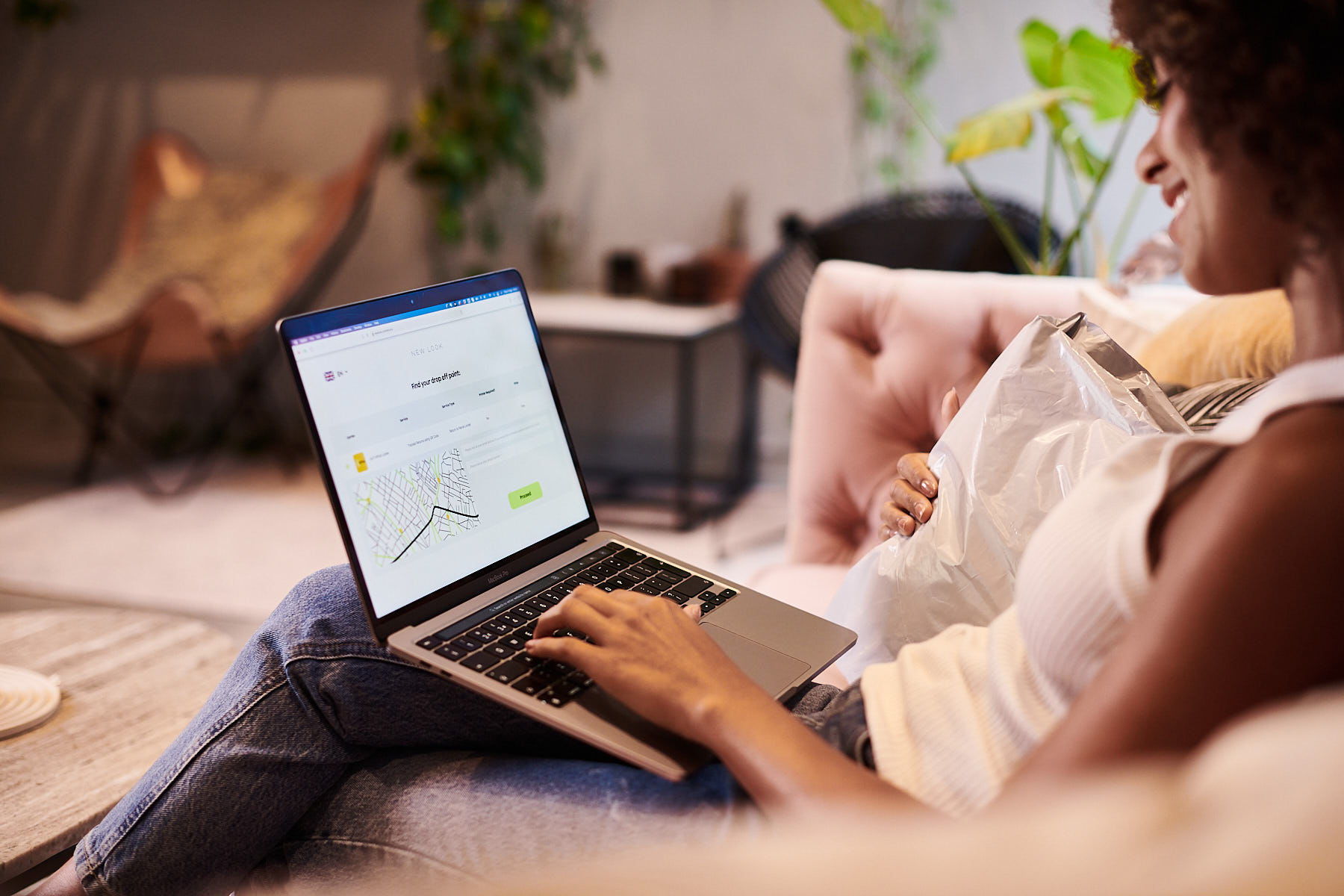
2. Transforming Customer Service with AI Chatbots
The rise of gen-AI has unlocked a significant advancement with the ability to integrate generative AI chatbots to handle common customer inquiries and post-purchase interactions.
Common customer queries like "how to return my item”, “do I need to print my label?”, “packaging guidelines”, “where is my return (WISMR)” and issues around return labels being out-of-date dominate customer service interactions. Of course, enquiries like this will naturally be reduced by an award-winning solution that provides proactive tracking updates throughout the returns process, however, there will always be eager and inquisitive customers ready to pester your Customer Service Team.
Chatbots with generative AI and automation workflows handling in the returns process can manage these inquiries efficiently in real-time, providing the customer with an improved fast contact experience. In fact, Chatbots can reduce customer service enquiries by as much as 30%. This allows the Customer Support team to focus more on complex customer interactions reducing operational costs and enhancing overall efficiency. In combination, Gen AI will support improving the customer experience and drive improved customer loyalty.
The future holds AI-powered customer assistants, that can respond to text or voice prompts, across various languages, to provide accurate responses to the customer's core needs, whilst maintaining the brand's tone of voice.
3. Predictive Inventory Management to Streamline Operations and Reduce Costs
Predicting product demand, with the additional complication of international returns flowing back in from various carriers is a significant challenge.
AI algorithms can predict product volumes by analysing large historical datasets to build out smart and accurate estimations on stock processing and replenishment times. By aligning these inventories closely to accurate predictions, retailers can reduce the frequency of markdowns and promotions needed to clear excess stock.
Particularly in industries with a short product lifecycle. Fast fashion items can be heading to landfill in as little as 6 weeks after their introduction to stores, with SHEIN releasing an astounding 10,000 new designs a day. Therefore, with designs getting so little time to be bought and shipped before they are already out-of-fashion, lengthy return times can put a real dent in their lifecycle. AI-driven inventory management is critical to achieving profitability through reduced stock write-down and additional warehouse and processing costs.
The future will allow for accurate predicted forecast volumes that give predict restock times, with automated workflow handling to secondary markets (donation, marketplace selling, etc.) depending on the product grade and market demand at the time of the return.
Did you know?
Second-hand and rental clothing markets are exploding. Successfully moving stock through trusted, in-country marketplaces can really impact a retailer’s bottom line. In fact, many retailers are already taking advantage of the resale market, contributing to its growth that is currently tracking 11 times faster than the broader retail clothing sector. ZigZag’s data also shows around half of consumers aged between 18 to 35 have recently bought or are planning to buy second-hand clothing.
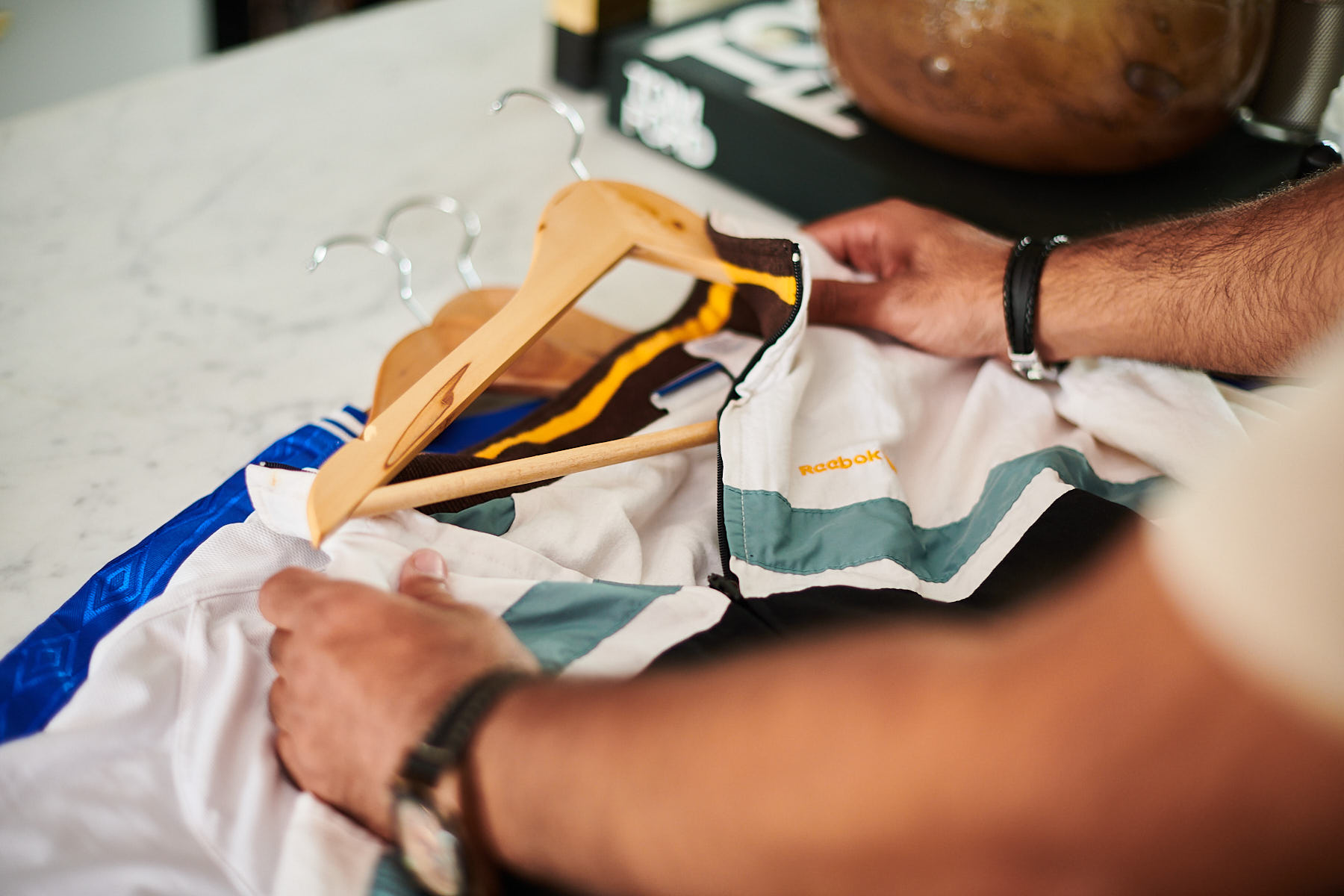
4. Accurate Delivery Date Estimations and Workflow Automations
Accurate predictive estimated delivery date (EDD) models are key to setting the stage for a good customer experience, reducing the likelihood of frustration from “Where is my Order/Return?” queries.
Predictive models are now considering multiple factors to decide whether parcels are likely to arrive late or early. These models can factor carrier performance data, historical shipping data, retail data, seasonal factors, and real-time logistics patterns. These models are adept at handling complex, multi-leg (often international) routes that give an accurate estimate that can reliably inform the customer, which can be done through email or SMS updates.
Embedding these estimated delivery date into post-purchase communications to update the customers, particularly on delays and in real time, improves the customer satisfaction, promotes the building of trust, and reduces Customer Service contacts and the associated overhead costs to handle.
In future, the industry will likely move to further embed the estimated delivery date to further personalise post-purchase communications, with customisable thresholds to adjust to often dynamic market conditions that can reduce the accuracy of EDD models. Through our parent company Global Blue, ZigZag has a partnership Shipup, a post-purchase experience expert that has already made some exciting developments in this area.
5. Advanced Returned Products Optimisation
Returns are a challenge and opportunity in any business. Optimising against optimisable return issues that cause a poor purchasing experience for the customer, brings improved CSAT, future repurchase rates, increased CLTV, and reduced return refund costs.
Smart analysis for strategic improvements that integrates generative AI technologies are starting to enable the ability to delve deep into the data, for fast identification of the root causes behind spikes or high return rates. AI-driven insights allow users intelligent and swift insights through automated trend analysis, outlier analysis, and cross-correlation analysis.
Applying the correct action to mitigate the spike of returns or high return rate will materially reduce unnecessary returns and associated warehousing and processing costs. Secondly, the impact of optimising avoidable pain points in real time, such as for product sizing, not as described as on the website and faulty return reasons, will improve customer satisfaction and loyalty. 77% of all revenue lost in 2023 by ZigZag’s retailers during the returns process originated from sizing issues. Whilst there will always be a level of size-related returns, through AI-driven insights, the biggest offending products with incorrect sizing can be tackled before they become larger issues.
In future, further additional context on benchmarks and industry behaviours in the machine learning models, will allow for an extra level of depth to understanding with each type of analysis, to enable the retailer to action recommendations with the highest level of confidence that the spike or issue is a genuine one to action with the right remedy.
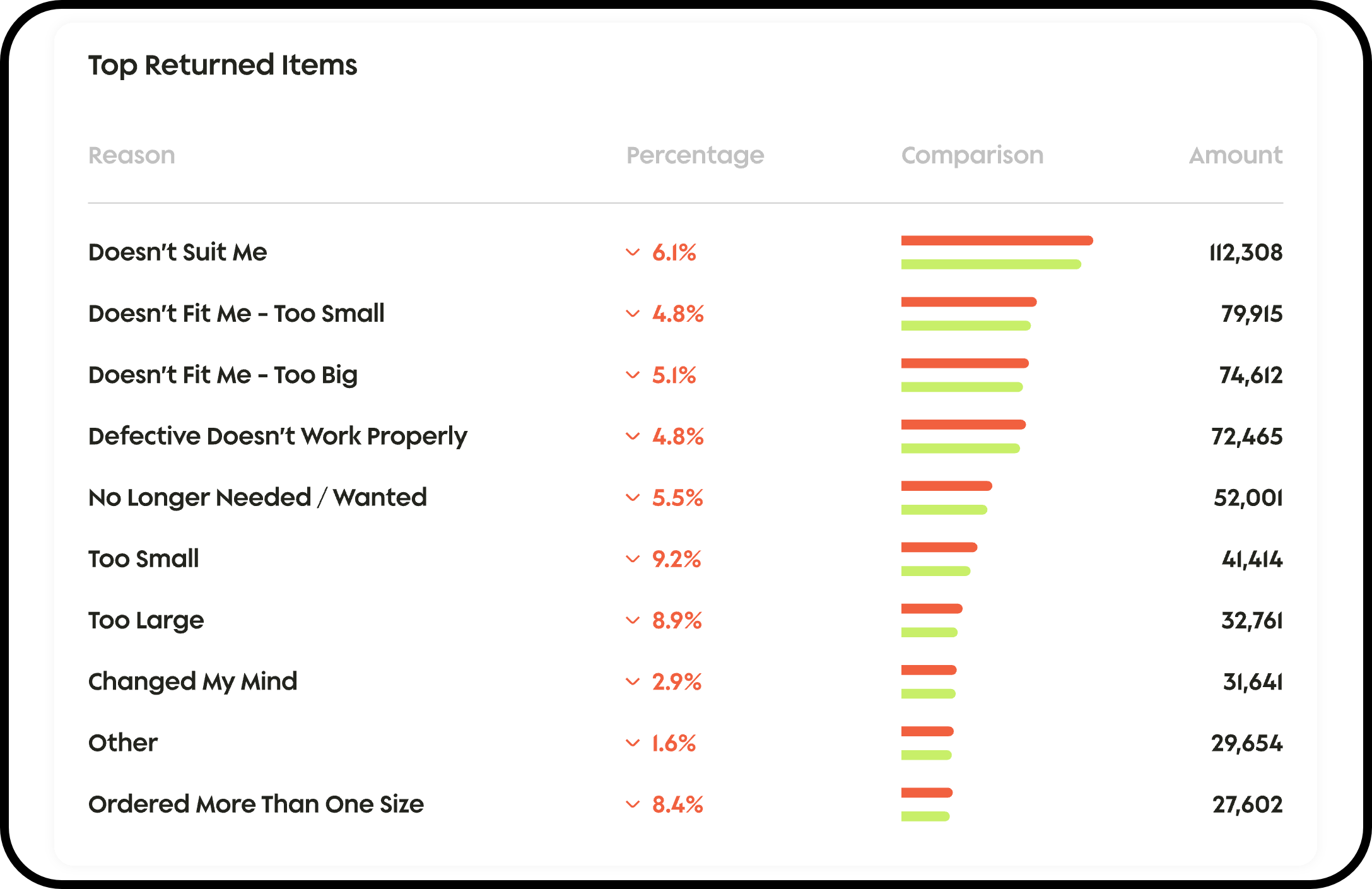
6. Personalised Product Upsell Recommendations
When customers return, it is not only an opportunity to correct an existing issue, but it is an opportunity to offer them a better product for their needs. Often customers return due to sizing, item not as described, not to my liking, or product quality issues.
Machine learning algorithms can now analyse the shopper's behaviour and preferences to suggest alternatives that may better suit their needs. This often includes:
- Similar Items – if the size was too small, the platform can show the larger available existing or similar styled products, and vice versa
- New Arrivals – showcasing new products that align to their tastes and needs
- Best Sellers – Highlighting the most popular products in the same grouping
- Similar Brands – Offering similar products but from a variety of alternative similar brands
These algorithms don’t just save the sale, it often adds new revenue generation from the customer, whilst offering a more intuitive exchange experience that boosts customer loyalty.
The future holds hyper-relevant tailored recommendations to the returning customer based off their historical preferences, to anticipate accurately the customer’s returning intent and future needs.
7. Fraud Detection and Handling
Returns fraud is a major cause of loss for many retailers, particularly for retailers that transact high value goods.
The ability to protect against fraud whilst maintaining transparency so that fraud flagged incidents are handled fairly and with appropriate communications.
Real time applications of machine learning models that trained to run on large historical databases are now being used to identify unusual customer patterns or deviations from normal customer behaviours to protect against fraud risk.
Fraudulent behaviours often include:
- Excessive returns – particularly when the goods are high value and numerous
- Same day returns – often when the tags have been found to have been removed (often product swapping for fakes)
- Identical defective returns – where it is hard to diagnose the underlying issue
- Renting or ‘wardrobing’ returners – who often abuse a free returns policy, and often wear high-value goods for a specific event
- Staging – often where customers purchase luxury goods to “show them off” on social media before returning them for a full refund.
- Damaged or used products – where customers can abuse a long returns window policy and return a worn used good that cannot be resold as new again.
Embedding these models into automated real-time workflows allows retailers to de-risk and prevent expensive new fraudulent behaviours, through triggering a manual touchpoint or review step.
The future holds combining automated grading, ML fraud models, and risk management workflows that effectively handle fraud incidences with transparent automated communications. This combination will result in reduced fraudulent revenue loss risks and protect the integrity of legitimate returners.
Ultimately, it’s imperative retailers don’t get too strict with challenging and punishing fraudulent returners. Top customers that buy a lot online will naturally return a lot too. So, make sure you are asking your systems to measure the correct fraudulent behaviour, and be sure to consult your returns management solution’s experts first.
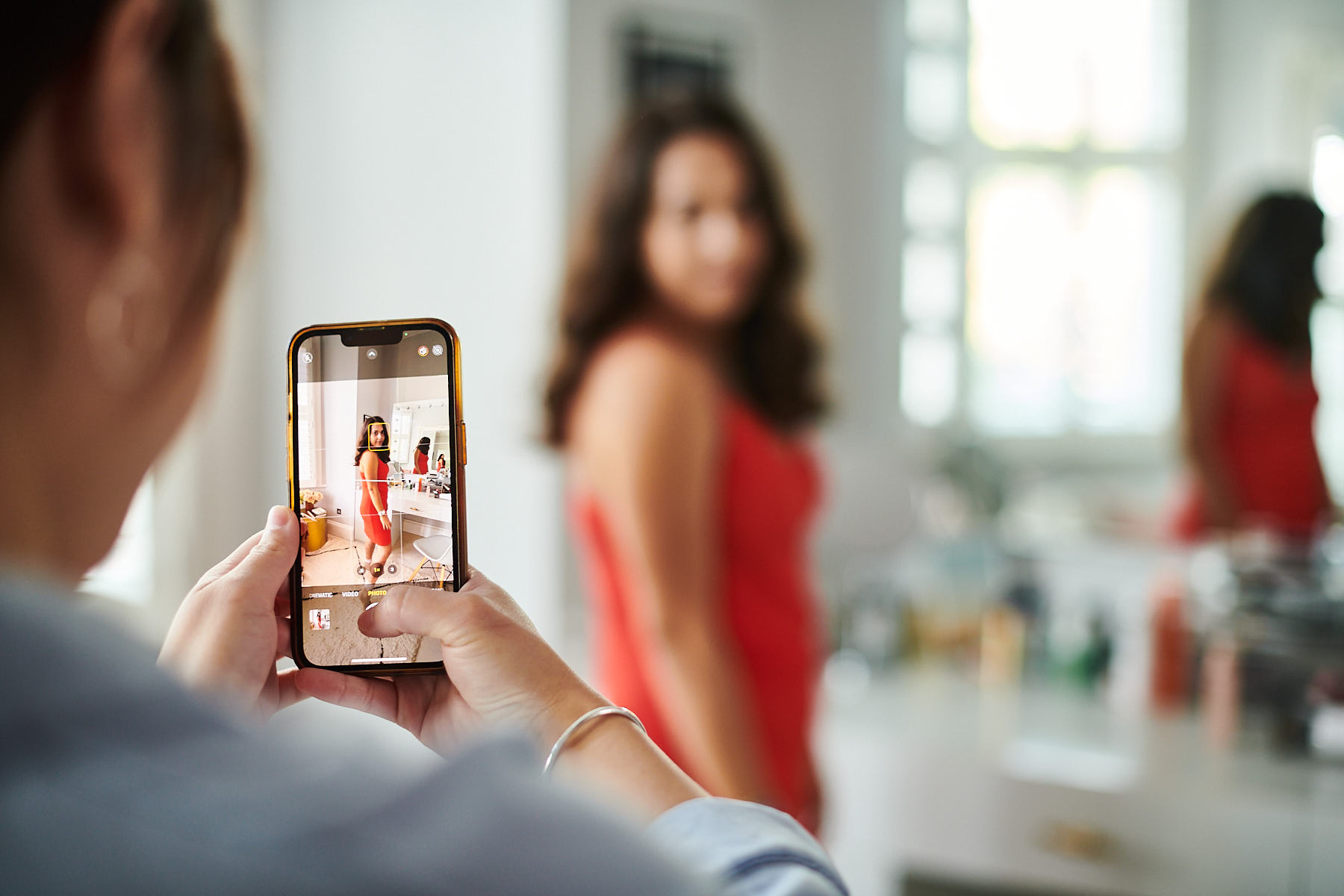
Recommendations
Speak to us to learn more about the top industry trends with AI and machine learning, and learn on how ZigZag offers smarter returns, and how ZigZag can help you transform your returns process into a strategic opportunity for you to get ahead of the curve, drive improved revenue, and grow your business.
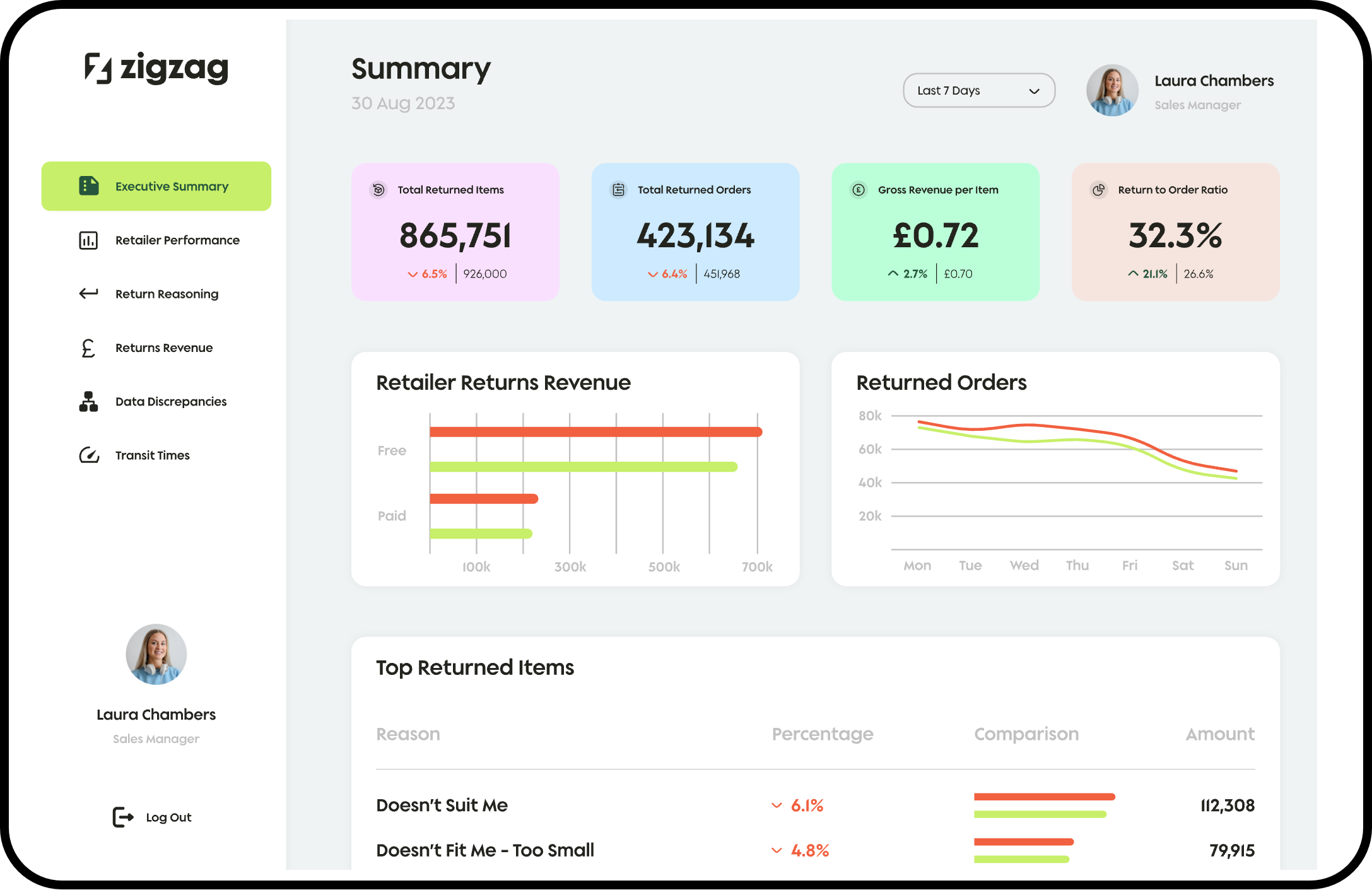